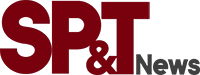
Features
Video Surveillance Week
Where AI-based video analytics work best
June 20, 2022 By Kai Moncino

Video analytics are an essential tool for perimeter security operations.
Motion-based as well as artificial intelligence (AI) and machine learning-enabled intrusion detection technologies have seen widespread adoption across airports, oil and gas facilities and other critical infrastructure locations throughout Canada. Today, new improvements are enhancing the classification accuracy of video analytics, enabling faster threat recognition and earlier intervention for crime deterrence.
The emergence of video analytics
For the first few decades of its implementation, analogue video cameras were the primary technology used to surveil facilities. These devices gave security personnel the ability to monitor an area to serve as an additional tool to on-site security guards.
In the early 2000s, the introduction of video analytics created a paradigm shift in the security industry. This technology used pixel-motion-based detection that triggered alarms based on a percentage of pixels that changed on a screen within defined borders; this enabled personnel to run motion detection algorithms using onboard hardware to expand the functionality of analogue video cameras.
No longer did an enterprise need to rely on the eyes of a security guard to catch suspicious activity — perimeter systems outfitted with video analytics could still deliver automated, instantaneous detection alerts. This ultimately increased the efficiency of monitoring and video operations, but common sources of motion such as wind-blown foliage and small animals oftentimes overwhelmed security operators with more false alarms than they could handle.
The state of AI-based analytics
Today, security directors require more from their perimeter intrusion detection systems (PIDS). As threats to critical infrastructure have evolved, it is no longer enough to detect movement at the perimeter. Security managers require analytics that reliably detect and classify an intruder, determine its location, and eliminate most false positives. Convolutional neural networks (CNN)-based analytics is an emerging technology that directly addresses this need. Built to replicate neurobiological systems to detect and classify objects in an image, convolutional systems rely on object classification to detect, classify and track a potential threat, improving video analytics precision, classification accuracy, geolocation of targets and resilience against false alarms.
How CNN video analytics work
To accomplish this, development engineers manually analyze thousands of images to determine the location and classification of interesting objects and train the CNN model on what is noteworthy and what should be ignored. While some CNN-based systems use public datasets to develop its classification capabilities, industry-leading CNN-based video analytics systems use datasets that are curated for specific security applications to reduce false alarms and maximize detection rate.
False Alarm Reduction: One of the most valuable advantages of CNN-based video analytics is their ability to reduce false alarms. Because CNN analytics do not use motion for input, they are less prone to sources of noise such as swaying foliage, camera shake and animals moving across the frame. By the numbers, CNN-based analytics have been shown to reduce false alarms by as much as 60 per cent, in a sample of 100 unique scenarios.
Robust Classification: Beyond false alarm reduction, CNN-based video analytics draw from libraries of thousands of images to deliver intelligent and informed intrusion detection alerts. Not only have these systems been tested to detect 15 per cent more threats than motion-based analytics in a sample of 100 unique scenarios, but they also improve on motion-based systems by classifying threats that may be slightly obscured or challenging to identify.
Because CNN-based video analytics do require more pixels on target than motion-based analytics, they are limited to approximately 20 per cent shorter classification ranges compared to motion-based analytics. This must be accounted for when designing the perimeter security solution and can be compensated for by selecting narrower lenses than with the equivalent motion-based analytic camera.
Where video analytics work best
For any of Canada’s critical infrastructure — whether an airport, an oil refinery, a data centre, or a power plant — CNN-based video analytics offer several benefits for perimeter protection and operational efficiency. To help understand the applications CNN-based video analytics would perform well in, explore the following use case examples:
Airports: For airports whose tarmacs are high-traffic areas, such as the Toronto Pearson International Airport, which served a total of 50,499,431 passengers in 2019, intruder classification and false alarm reduction, along the perimeter is indispensable. CNN-based video analytics represent a turnkey solution for large airports, where reducing surveillance video noise can allow security personnel to focus energy and resources on priority alerts.
Oil & gas refinery: With the third-largest oil reserves in the world, Canada’s oil and gas facilities house assets vital to producing energy. From the oil well to the refinery, CNN-based video analytics empower security personnel to provide greater detection and classification accuracy in every environment, accurate target tracking, and expanded situational awareness.
Substations: As reported by CIGRE, 88 per cent of substations experience at least one break-in every year. For electrical utilities whose rural substations may have no full-time security staff on-site, accurate classification can be the difference between a preventable perimeter intrusion and a prevented one. CNN-based video analytics enable security personnel, on- or off-site, to alert first responders and inform the best possible response.
Data centres: In 2021, the global cloud data centre traffic grew to 20.6 zettabytes (ZB), according to Cisco. That same year, Cisco expected to witness the number of hyperscale data centres grow from 228 in 2016 to 628. As more industries migrate their highly sensitive data to data centres, data centre perimeter security has become more important than ever. CNN-based video analytics allow data centre security personnel to expand their situational awareness, and in turn, sharpen perimeter security responsiveness.
Regardless of the perimeter, fewer false alarms, greater detection and classification accuracy, expanded situational awareness and novel abilities to track and respond to intruders in real time delivers security personnel an invaluable tool. Designed for continuous evolution, CNN-based analytics are built to keep pace with future technological developments and ever-evolving threats, establishing them as a solution that is truly future-proofed.
Kai Moncino is the director of global business development, security at Teledyne FLIR (www.flir.ca).
Print this page